Exclusive Interview: Rossum – AI for Documents
Rossum is a deep-tech startup that joined StartupYard with an understanding of the technology they were developing, but no clear use-case for that technology. The team, a group of PHD candidates in artificial intelligence and machine learning, were exploring applications for machine learning in routine tasks like image categorization.
Through the acceleration process, the Rossum team found opportunities in applying machine learning to corporate accounting, where automation technology has failed to make serious gains in productivity in recent years, due to the sheer complexity and variability of financial documentation. Even invoices alone, of which over 1 billion are sent each day worldwide, are highly resistant to automation due to technical limitations imposed due to historical factors. Rossum began to apply their technology to this problem, and have already made major breakthroughs with the help of a few early key partners.
I sat down this week with Co-Founders Tomas Gogar, and Petr Baudis to talk about Rossum:
Hi Tom, tell us a bit about Rossum. Where did the idea come from?
Tomas Gogar: Hi! The idea for Rossum dates back several years, and it started out as something very different. Our team is a group of PHD candidates who have been studying AI/Machine learning and semantic understanding for a long time.
My Co-Founder, Petr Baudis, is quite well known in AI circles for developing the leading open-source Question Answering AI, something similar to IBM’s own Watson. We actually know that some members of the IBM team periodically test his platform to see how it performs.
Petr also laid the groundwork for Google’s AlphaGo project (the AI that beat a world master in the game Go, many years before it had been predicted that a computer could beat a human). Google cited Petr in their landmark paper in the journal Nature.
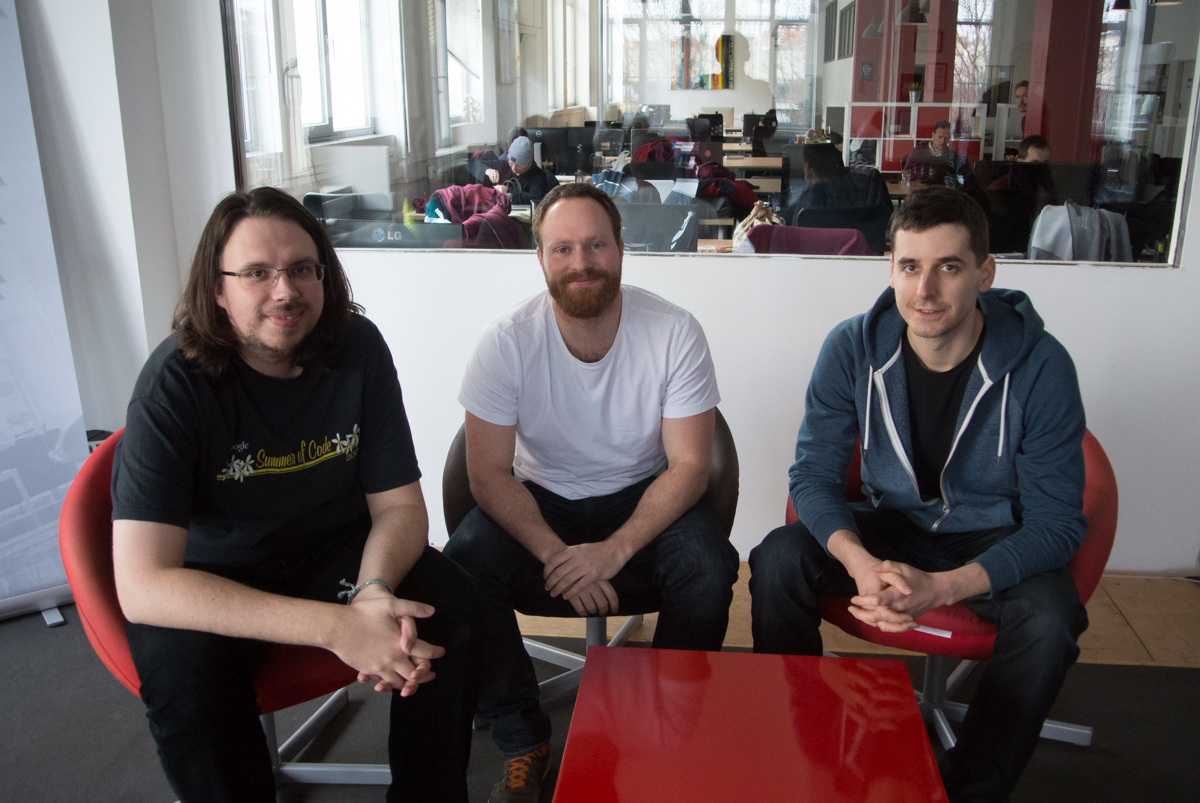
The Rossum Team: Petr Baudis (left), and Tomas Gogar (middle), with Tomas Tunys (right)
I also have published groundbreaking research on the use of neural nets to parse and understand complex semantics in written text (such as semi-structured documents). It was actually this research that led us to the underlying idea behind Rossum.
Like everybody in this field, we’ve been fascinated by how neural networks and machine learning can be applied to massive volumes of data online. We started with a very popular objective, which is recognition of images and their contents, commonly called computer vision.
When we applied to StartupYard, we had the idea of providing a kind of “AI as a Service,” platform that could be used by researchers or data scientists to apply machine learning to their own problems. One of our innovations was being able to train a neural network on very limited data, which made it very useful for this purpose.
In mentoring though, we quickly discovered that there is actually a huge need for AI that understands and can parse text, in many different formats. We had assumed that problem had been solved by OCR (Optical Character Recognition), but in fact automation based on OCR alone has not really improved in effectiveness in many years. It remains a very hit and miss process.
You’re solving what seems like an unlikely problem- processing invoices. How can AI be applied to that problem?
Yes, it seemed unlikely to us as well! But what we found when we met with mentors from the big 4 accounting firms is that, even in 2017, invoice handling is largely still a manual process. This is despite OCR being around for 25 years already, and electronic invoices existing for over 50 years.
That’s mainly because despite all attempts to standardize and streamline invoicing (including newer techniques like using QR codes to allow machines to read them), it remains a problem that is really too big for any one player to solve. No government or company has been able to get enough others to agree on a single standard, and that has meant, effectively, that the complexity of processing invoices has not changed much for decades. It may actually have gotten worse, thanks to the increasing complexity of the products and services for which we receive invoices, and the increase in the total volume of invoices.
So just imagine you are an accounting company. You receive thousands of invoices a day. Maybe hundreds of thousands a year. If every invoice takes your accounting people just one minute to process and confirm, that’s hundreds of hours of work that needs to be done. And that is aside from the possibility that you are audited, and have to check all that work for a second time. The human error rate for invoice processing is low, but mistakes are very expensive. Fraud is surprisingly common as well, as bad actors take advantage of the complexities of billing to trick people into paying for non-existent things.
So we began to realize that one answer to this seemingly intractable problem was AI. The big hurdle for automating invoice processing is that no two invoices are ever exactly the same. Getting a machine to recognize one type of invoice, and correctly pull the right information out of it is non-trivial, but certainly possible with existing technology. The problem is that at any time, companies are receiving invoices from many, many parties, a company can change the way it formats invoices, or an invoice can contain a mistake or can be fraudulent.
So that means that no matter how streamlined your process may be, it still requires human-level judgement to confirm that everything is correct. That creates a bottleneck, because human-level judgement takes a long time to apply. People are very good at semantics, but we are also very slow. And you have the influence of many human factors: being tired, misreading a number, forgetting to double check.
Rossum: Human level judgement is slow. And most AI is dumb. Read the exclusive interview: Share on X
AI that can read and understand an invoice at a human level can also be made to do the same work many, many times faster than a human. So if you train a neural network to be able to recognize and work with invoices it has never seen before (just as a human can do), then you can turn hundreds of hours of tedious work into a matter of a few seconds or minutes of computer processing time.
Who would use this technology? Why hasn’t it been developed already?
Well, neural networks are really just starting to be applied to these problems. One of the reasons this hasn’t been done already is that processing power and computer architecture hasn’t been powerful enough to make it possible. The other side of that equation is the data itself. Until recently, much of the data needed to train a neural network didn’t exist in a form that a network could actually handle. Invoices were on paper, or they were “electronic” and thus in a form that could be handled automatically by a hard-wired program.
In the past decade though, the volume of data that we can apply to machine learning has exploded. That’s why AI and machine learning have suddenly become hot topics again.
The other issue has been that the specific methodologies for training and checking the accuracy of neural networks have been evolving, and are just starting to become really useful for this kind of work. You can just show a million invoices to a neural network, but getting it to focus on what is important is not something you can just ask for. You have to be able to train the algorithms in ways that help it eliminate useless information and focus on what you want it to focus on.
The process is analogous to the way a baby learns. When a baby is born, its ability to sense information around it is very limited. It can’t see or hear very well, and it can’t process what it does see or hear. Slowly it becomes more able to sense information, and it begins to use that new information to construct an understanding of the world around it. Then comes language: the way that a human mind is able to abstract information and complexity, and imagine new things it has never seen before.
If we are to use this analogy, today neural networks are operating mostly blind, and with little understanding of language to create or understand abstract ideas. As we expose them to more information, we also have to teach them a “language” that they can use to extract something useful from that data. Rossum is a part of that language: we are helping neural networks to understand what we want them to do, and why.
The answer to who would use this technology, is, well, everyone! Human level judgement in understanding documents, even of only a very specific type, would save huge amounts of routine work for humans, who can spend that time doing things that are more natural to them. There is nothing natural for a person about spending their days processing invoices. We can learn it, but we never love to do it.
If you consider how many of these kinds of tasks exist, you realize that we spend massive amounts of time doing things that just don’t bring us anything of value, and in fact waste our time and demoralize us. That is the promise of AI in the near future: this ability to free people from having to deal with things that we just don’t get anything out of, but that just have to be done by someone.
What will be your near-term strategy for bringing Rossum’s technology to market?
As mentioned, we are already in contact with representatives of the major accounting firms. They have the biggest immediate need for Rossum, and they also have the data that Rossum needs to be able to train itself and understand invoices it has never seen before.
In the next few years, we want to have a platform that can understand and work with invoices from literally anywhere in the world, many times faster than a human can, with an error-rate lower than any human. Then the work becomes helping these companies to implement the solution, and find ways for Rossum to interact with other systems so that it can help companies streamline their document handling operations.
It is not much use to an accounting firm for an AI to understand all its invoices, if the AI doesn’t also know how to connect with their other systems, and give the outputs they need to take action. That in itself will be a challenge, and one we anticipate will take some time and development. Still, once you have the ability to process invoices with human or above-human accuracy and speed, then there is a huge incentive on the part of companies to integrate that solution into their systems.
We believe that Rossum will be a must-have for accounting firms in the very near future. And once that value has been clearly demonstrated, we can apply the technology to many other processes that are similar in nature. Auditing, analysis and processing of other documents, etc. Rossum could be a backbone for a suite of intelligent applications that takes care of a wide range of tasks that are complex, and repetitive.
We also want to open up Rossum as an online platform, to allow small and medium sized companies to find it and gain the same value from it. Currently, invoice processing services online come at a heavy cost- over a Euro per invoice in many cases. Rossum can do the same work for a tiny fraction of those costs, and it can do it instantaneously, with a very high degree of certainty.
An automatic platform solution is faster, cheaper, and safer for companies that have confidential information they don’t want others to have access to.
How has your experience been at StartupYard? What surprised you?
Petr Baudis: We were rather hesitant about joining StartupYard actually, even though we received several personal recommendations from some of the top alumni founders. We were thinking “hey, we have an office and our own good network of contracts, does StartupYard make sense for us?” and we applied at the last minute and very tentatively.
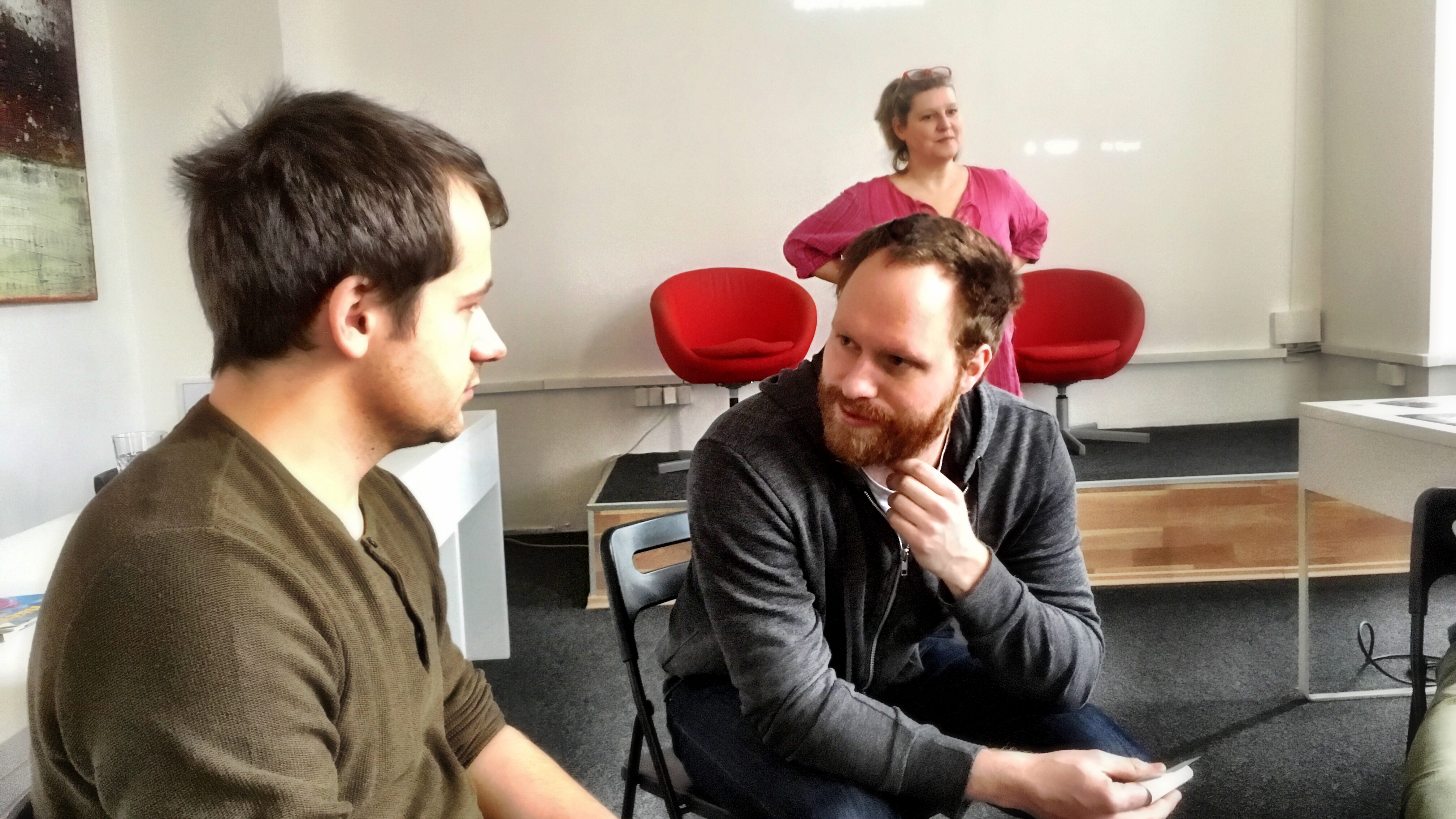
Tomas Gogar (right), works with another co-founder at the StartupYard voice workshop
Oh boy, we were in for a real ride when we did decide to join. Mentoring sessions gave us a much wider scale of perspectives than we could ever gain from our own professional network, and a real and much needed shift of focus from the technical to business. That we expected a little – but it surprised us how eager the core StartupYard team was to help with their experience and feedback, these few people (including you, Lloyd!) really became an important part of Rossum’s story.
And most importantly, StartupYard finally gave us the impulse to really focus on one single thing – we were busy people before, but now we had the reason to finally drop all the side projects for good. We thought the first mentoring month would be the most intense phase, but the pace is only picking up since, and without the “little” pushing by the StartupYard team we would be much more comfortable, getting a good eight hours of sleep a night, but still at the beginning.