Exclusive Interview: Neuron Soundware Wins Yet Another Award
Neuron Soundware: Winning Awards and Customers
Since leaving StartupYard in this year, Neuron Soundware has made “soundwaves” in the startup community in Europe, winning multiple awards, including Vodafone’s Idea of the Year, and now, this week, Ceska Sporitelna’s Startup of the Year.
The company has come a long way in a year– from a small team that was able to demonstrate, at SY Demo Day 2016, a machine learning algorithm that could learn to mimic a human actor, to a company that provides machine learning diagnostic software to large equipment operators. They’ve received considerable press coverage. Already, they count both Siemens and Deutsche Bahn among their customers.
I caught up with Pavel Konecny, Co-Founder and CEO of Neuron Soundware, to talk about what the team has been through since leaving StartupYard, and where they’re going in the near future:
Hi Pavel, a lot has happened for Neuron Soundware since you left StartupYard. Can you tell us what you’ve been up to since the program?
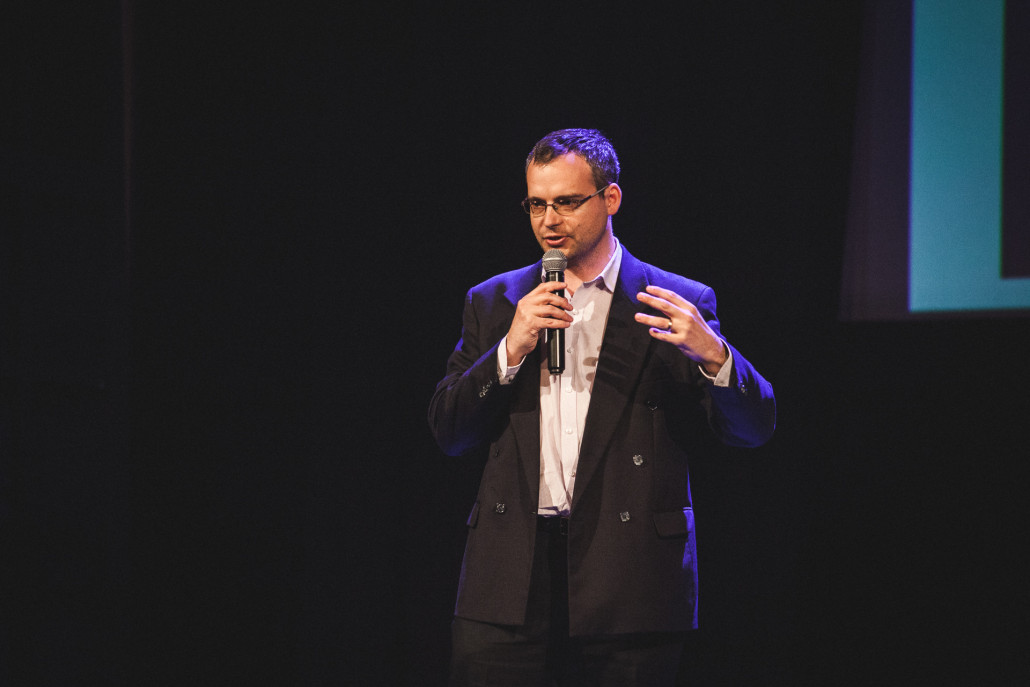
Pavel Konecny, of NeuronSoundware, talks about machine learning and sound.
We were very busy of course. We have presented Neuron Soundware at international startup and advance engineering conferences in US, UK, Germany and Czech Republic. We got a lot of contacts, which we are going to leverage. We are also proud that we found our first paying customers including companies such as Siemens and Deutsche Bahn.
What are you providing for those new customers?
We provide sound analytics algorithms as a service – an early warning of the coming mechanical issues of machines such as wind turbines, escalators, etc.
Towards the end of StartupYard 2016, your team decided to focus on diagnosing mechanical issues for machinery. Can you tell us a bit more about how this works?
Complex machinery with moving parts always has multiple points of potential failure. There are basically two ways to solve that issue: either you wait until something breaks, or you proactively monitor the parts you know are likely to break, and fix them before they do.
Waiting for a failure can be expensive, and even dangerous. We can’t wait for an airplane engine to just stop working. You can’t have a printing press suddenly fail an hour before the trucks arrive. The loss in business alone makes it a major vulnerability.
Why can’t humans do this kind of work? Why is a machine more effective?
I’ll give you a real world example: just google “failed wind turbine”. You would find scores of different pictures and videos from all over the world. Wind turbines are giant and very fast moving machines. If the blade breaks a part in the full speed, you can find the pieces miles away and this can be quite dangerous. Preventing these events is a huge challenge.
Currently they do exhaustive physical checks. What we found was that sound, the sound of a machinery operating normally, or machinery nearing a failure, was a very important source of data that was not being employed fully.
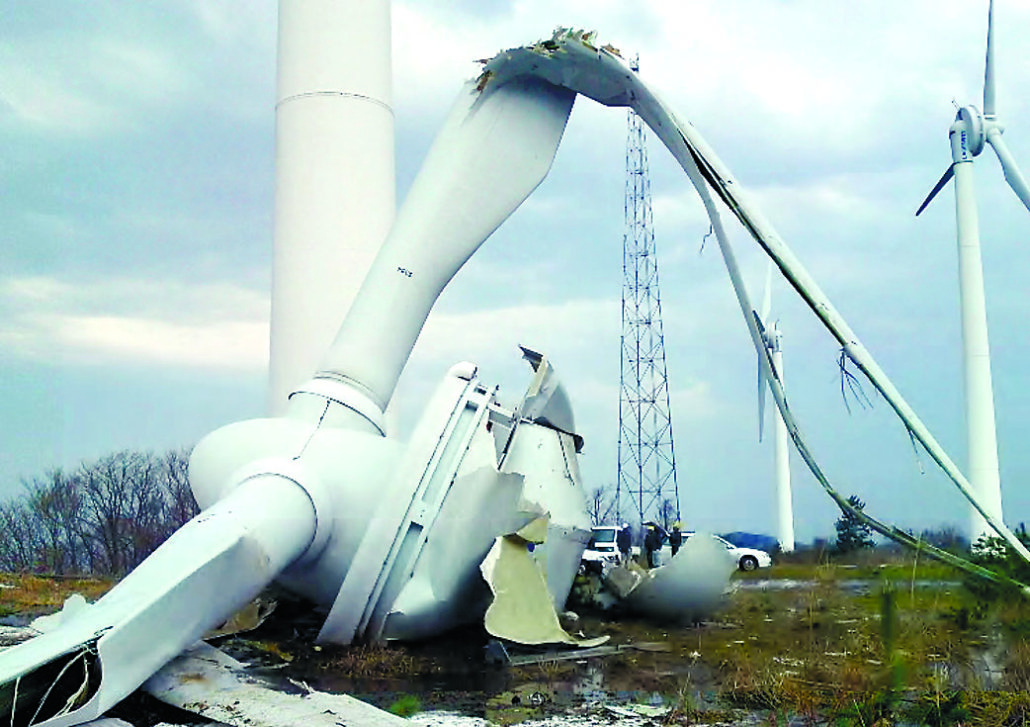
Photo Courtesy of Kyoto Prefecture, Japan
If you can understand a machine by the sounds it produces, you can reduce the risk of sudden failures, and increase the effectiveness of maintenance, since repairs are directed according to some available data about what’s working and what isn’t.
A machine learning algorithm can learn to connect data points that a human would ignore. A particular sound or a particular frequency may lead to a particular failure at a higher rate. Many of these tasks are above the capability of a human, who has a limited attention span, and limited memory.
There are also practical ways in which a machine is more effective: nobody can listen inside an airplane engine while it’s flying. Nobody can consistently diagnose a mechanical failure based on auditory clues that humans can’t actually detect. You need machines and machine learning for that, and that’s the breakthrough we’ve made.
How does Neuron Soundware learn?
Some issues can be simulated and some just appear time to time and you need to be ready to record them.
Hence we have developed our IoT device equipped with several types of microphones, which we use for the initial data collection. The device is mounted to the machines, continuously listening and transferring audio files to our central server. When we collect enough samples, we use them as an input to our learning algorithm. The machine health monitoring is done using the same IoT device.
You’ve now conducted some pilots as well, how was the experience, and what have you learned that surprised you and your team?
We were surprised several times of the effectiveness of deep learning technology. It works with all type of sounds. If we collect enough samples, we can achieve quality of recognition above 99.5%. And that would get even better as the system would collect more data.
Already, our approach can detect and diagnose mechanical faults that human diagnosticians cannot.
What has been Neuron Soundware’s biggest challenge since leaving StartupYard?
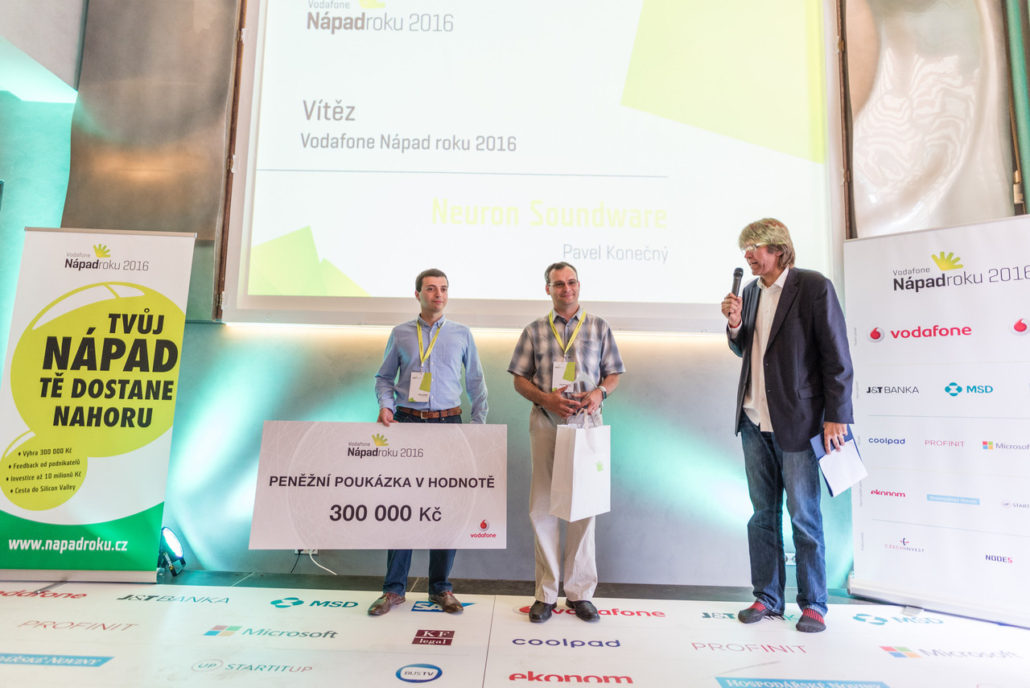
The Neuron Soundware team wins Vodafon’s Idea of the Year
We are travelling a lot. So the most of the communication happens via Slack and Hangouts. We meet in-person as the whole team only once or twice a week. That’s an intense time, when we need to sort-out a lot of items quickly. It was very refreshing, when (Co-Founder) Filip got married in October and we were all together and not discussing business matters. So we went to (3rd Co-founder) Pavel’s band’s concert last weekend as keeping friendly team spirit is very important to us.
You recently recommended another deep-tech startup for our program. Why did you recommend StartupYard? What do you think has been the most positive outcome of acceleration for your team?
We would not be where we are now, without StartupYard. We started with a long list of ideas, where to apply AI technology, and we end-up with The idea of the Year (awarded by Vodafone Foundation)- and now Startup of the Year (from Ceska Sporitelna).
So we would like to thank again the many mentors we met during the first month of the program. It also changed our mindset in several ways: how to validate the business potential; how to pitch our product. Rather talk to people than flood them with documentation.
I used to start a meeting by passing out a complicated document, outlining everything I wanted people to know. What I learned along the way is that it’s equally important for people to get to know me and my team as people. Business is about making a personal connection- and that was an important lesson.
You’ve been talking with investors recently. What have you discovered during this process? What are you planning to do with the funds when you raise them?
It takes much longer than anticipated. They all stated how simple it is. It looks nice as starts with an interview, a short two page document. Then you follow with more meetings and committee board presentations, longer documents and the whole process of due diligence.
It is difficult to imagine, even for me, what we could be capable of doing in two or three years with our self-learning AI technology. And how much value and money we can make. We will use the investment to expand our business. With a larger development team, we could quicker complete the self-service sound analytics platform we are working on. That would make our business highly scalable and we could ramp-up our sales team.
Neuron Soundware’s core technology has a lot of interesting applications. Where do you see your team focusing its efforts within the next few years?
We are working on a way to combine effectively the different datasets we are collecting.
That would practically allow us to skip the phase of training as the neural network would be already pre-trained to recognize a wide set of potential issues. This is basically the way a human mind operates: you use past experiences to gain insight on new situations, even if they are very different. A machine can be taught to do the same thing, once given enough data.
The goal then, would be to start shipping a small smart IoT device in large volumes, ready to be used within any machine. Imagine a kind of silent digital mechanic, always sitting and monitoring complex equipment, all the time, and getting better, and better at the job every hour of every day. That’s really the future we are building with Neuron Soundware.